A monthly podcast where we discuss recent research and developments in the world of Neural Search, LLMs, RAG and Natural Language Processing with our co-hosts Jakub Zavrel (AI veteran and founder at Zeta Alpha) and Dinos Papakostas (AI Researcher at Zeta Alpha).
…
continue reading
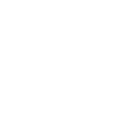
1
AGI vs ASI: The future of AI-supported decision making with Louis Rosenberg
54:42
54:42
Прослушать позже
Прослушать позже
Списки
Нравится
Нравится
54:42
In this episode of Neural Search Talks, we have invited Louis Rosenberg, CEO of Unanimous.AI, to discuss the future of AI in decision-making, contrasting the development of artificial superintelligence (ASI) with collective human intelligence systems, such as swarm intelligence. In particular, Louis argues that the advancement of AI should focus on…
…
continue reading
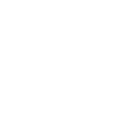
1
EXAONE 3.0: An Expert AI for Everyone (with Hyeongu Yun)
24:57
24:57
Прослушать позже
Прослушать позже
Списки
Нравится
Нравится
24:57
In this episode of Neural Search Talks, we welcome Hyeongu Yun from LG AI Research to discuss the newest addition to the EXAONE Universe: EXAONE 3.0. The model demonstrates strong capabilities in both English and Korean, excelling not only in real-world instruction-following scenarios but also achieving impressive results in math and coding benchma…
…
continue reading
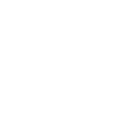
1
Zeta-Alpha-E5-Mistral: Finetuning LLMs for Retrieval (with Arthur Câmara)
19:35
19:35
Прослушать позже
Прослушать позже
Списки
Нравится
Нравится
19:35
In the 30th episode of Neural Search Talks, we have our very own Arthur Câmara, Senior Research Engineer at Zeta Alpha, presenting a 20-minute guide on how we fine-tune Large Language Models for effective text retrieval. Arthur discusses the common issues with embedding models in a general-purpose RAG pipeline, how to tackle the lack of retrieval-o…
…
continue reading
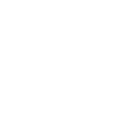
1
ColPali: Document Retrieval with Vision-Language Models only (with Manuel Faysse)
34:48
34:48
Прослушать позже
Прослушать позже
Списки
Нравится
Нравится
34:48
In this episode of Neural Search Talks, we're chatting with Manuel Faysse, a 2nd year PhD student from CentraleSupélec & Illuin Technology, who is the first author of the paper "ColPali: Efficient Document Retrieval with Vision Language Models". ColPali is making waves in the IR community as a simple but effective new take on embedding documents us…
…
continue reading
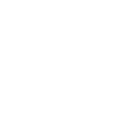
1
Using LLMs in Information Retrieval (w/ Ronak Pradeep)
22:15
22:15
Прослушать позже
Прослушать позже
Списки
Нравится
Нравится
22:15
In this episode of Neural Search Talks, we're chatting with Ronak Pradeep, a PhD student from the University of Waterloo, about his experience using LLMs in Information Retrieval, both as a backbone of ranking systems and for their end-to-end evaluation. Ronak analyzes the impact of the advancements in language models on the way we think about IR s…
…
continue reading
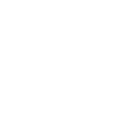
1
Designing Reliable AI Systems with DSPy (w/ Omar Khattab)
59:57
59:57
Прослушать позже
Прослушать позже
Списки
Нравится
Нравится
59:57
In this episode of Neural Search Talks, we're chatting with Omar Khattab, the author behind popular IR & LLM frameworks like ColBERT and DSPy. Omar describes the current state of using AI models in production systems, highlighting how thinking at the right level of abstraction with the right tools for optimization can deliver reliable solutions tha…
…
continue reading
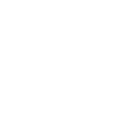
1
The Power of Noise (w/ Florin Cuconasu)
11:45
11:45
Прослушать позже
Прослушать позже
Списки
Нравится
Нравится
11:45
In this episode of Neural Search Talks, we're chatting with Florin Cuconasu, the first author of the paper "The Power of Noise", presented at SIGIR 2024. We discuss the current state of the field of Retrieval-Augmented Generation (RAG), and how LLMs interact with retrievers to power modern Generative AI applications, with Florin delivering practica…
…
continue reading
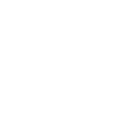
1
Benchmarking IR Models (w/ Nandan Thakur)
21:55
21:55
Прослушать позже
Прослушать позже
Списки
Нравится
Нравится
21:55
In this episode of Neural Search Talks, we're chatting with Nandan Thakur about the state of model evaluations in Information Retrieval. Nandan is the first author of the paper that introduced the BEIR benchmark, and since its publication in 2021, we've seen models try to hill-climb on the leaderboard, but also fail to outperform the BM25 baseline …
…
continue reading
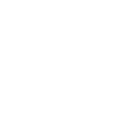
1
Baking the Future of Information Retrieval Models
27:05
27:05
Прослушать позже
Прослушать позже
Списки
Нравится
Нравится
27:05
In this episode of Neural Search Talks, we're chatting with Aamir Shakir from Mixed Bread AI, who shares his insights on starting a company that aims to make search smarter with AI. He details their approach to overcoming challenges in embedding models, touching on the significance of data diversity, novel loss functions, and the future of multilin…
…
continue reading
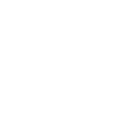
1
Hacking JIT Assembly to Build Exascale AI Infrastructure
38:04
38:04
Прослушать позже
Прослушать позже
Списки
Нравится
Нравится
38:04
Ash shares his journey from software development to pioneering in the AI infrastructure space with Unum. He discusses Unum's focus on unleashing the full potential of modern computers for AI, search, and database applications through efficient data processing and infrastructure. Highlighting Unum's technical achievements, including SIMD instruction…
…
continue reading
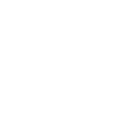
1
The Promise of Language Models for Search: Generative Information Retrieval
1:07:31
1:07:31
Прослушать позже
Прослушать позже
Списки
Нравится
Нравится
1:07:31
In this episode of Neural Search Talks, Andrew Yates (Assistant Prof at the University of Amsterdam) Sergi Castella (Analyst at Zeta Alpha), and Gabriel Bénédict (PhD student at the University of Amsterdam) discuss the prospect of using GPT-like models as a replacement for conventional search engines.Generative Information Retrieval (Gen IR) SIGIR …
…
continue reading
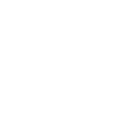
1
Task-aware Retrieval with Instructions
1:11:13
1:11:13
Прослушать позже
Прослушать позже
Списки
Нравится
Нравится
1:11:13
Andrew Yates (Assistant Prof at University of Amsterdam) and Sergi Castella (Analyst at Zeta Alpha) discuss the paper "Task-aware Retrieval with Instructions" by Akari Asai et al. This paper proposes to augment a conglomerate of existing retrieval and NLP datasets with natural language instructions (BERRI, Bank of Explicit RetRieval Instructions) a…
…
continue reading
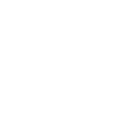
1
Generating Training Data with Large Language Models w/ Special Guest Marzieh Fadaee
1:16:14
1:16:14
Прослушать позже
Прослушать позже
Списки
Нравится
Нравится
1:16:14
Marzieh Fadaee — NLP Research Lead at Zeta Alpha — joins Andrew Yates and Sergi Castella to chat about her work in using large Language Models like GPT-3 to generate domain-specific training data for retrieval models with little-to-no human input. The two papers discussed are "InPars: Data Augmentation for Information Retrieval using Large Language…
…
continue reading
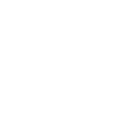
1
ColBERT + ColBERTv2: late interaction at a reasonable inference cost
57:30
57:30
Прослушать позже
Прослушать позже
Списки
Нравится
Нравится
57:30
Andrew Yates (Assistant Professor at the University of Amsterdam) and Sergi Castella (Analyst at Zeta Alpha) discus the two influential papers introducing ColBERT (from 2020) and ColBERT v2 (from 2022), which mainly propose a fast late interaction operation to achieve a performance close to full cross-encoders but at a more manageable computational…
…
continue reading
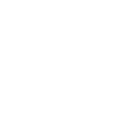
1
Evaluating Extrapolation Performance of Dense Retrieval: How does DR compare to cross encoders when it comes to generalization?
58:30
58:30
Прослушать позже
Прослушать позже
Списки
Нравится
Нравится
58:30
How much of the training and test sets in TREC or MS Marco overlap? Can we evaluate on different splits of the data to isolate the extrapolation performance? In this episode of Neural Information Retrieval Talks, Andrew Yates and Sergi Castella i Sapé discuss the paper "Evaluating Extrapolation Performance of Dense Retrieval" byJingtao Zhan, Xiaohu…
…
continue reading
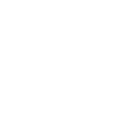
1
Open Pre-Trained Transformer Language Models (OPT): What does it take to train GPT-3?
47:12
47:12
Прослушать позже
Прослушать позже
Списки
Нравится
Нравится
47:12
Andrew Yates (Assistant Professor at the University of Amsterdam) and Sergi Castella i Sapé discuss the recent "Open Pre-trained Transformer (OPT) Language Models" from Meta AI (formerly Facebook). In this replication work, Meta developed and trained a 175 Billion parameter Transformer very similar to GPT-3 from OpenAI, documenting the process in d…
…
continue reading
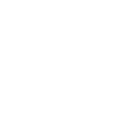
1
Few-Shot Conversational Dense Retrieval (ConvDR) w/ special guest Antonios Krasakis
1:23:11
1:23:11
Прослушать позже
Прослушать позже
Списки
Нравится
Нравится
1:23:11
We discuss Conversational Search with our usual cohosts Andrew Yates and Sergi Castella i Sapé; along with a special guest Antonios Minas Krasakis, PhD candidate at the University of Amsterdam. We center our discussion around the ConvDR paper: "Few-Shot Conversational Dense Retrieval" by Shi Yu et al. which was the first work to perform Conversatio…
…
continue reading
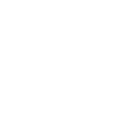
1
Transformer Memory as a Differentiable Search Index: memorizing thousands of random doc ids works!?
1:01:40
1:01:40
Прослушать позже
Прослушать позже
Списки
Нравится
Нравится
1:01:40
Andrew Yates and Sergi Castella discuss the paper titled "Transformer Memory as a Differentiable Search Index" by Yi Tay et al at Google. This work proposes a new approach to document retrieval in which document ids are memorized by a transformer during training (or "indexing") and for retrieval, a query is fed to the model, which then generates au…
…
continue reading
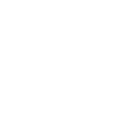
1
Learning to Retrieve Passages without Supervision: finally unsupervised Neural IR?
59:10
59:10
Прослушать позже
Прослушать позже
Списки
Нравится
Нравится
59:10
In this third episode of the Neural Information Retrieval Talks podcast, Andrew Yates and Sergi Castella discuss the paper "Learning to Retrieve Passages without Supervision" by Ori Ram et al. Despite the massive advances in Neural Information Retrieval in the past few years, statistical models still overperform neural models when no annotations ar…
…
continue reading
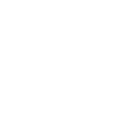
1
The Curse of Dense Low-Dimensional Information Retrieval for Large Index Sizes
54:13
54:13
Прослушать позже
Прослушать позже
Списки
Нравится
Нравится
54:13
We discuss the Information Retrieval publication "The Curse of Dense Low-Dimensional Information Retrieval for Large Index Sizes" by Nils Reimers and Iryna Gurevych, which explores how Dense Passage Retrieval performance degrades as the index size varies and how it compares to traditional sparse or keyword-based methods. Timestamps: 00:00 Co-host i…
…
continue reading
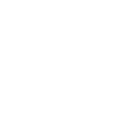
1
Shallow Pooling for Sparse Labels: the shortcomings of MS MARCO
1:07:17
1:07:17
Прослушать позже
Прослушать позже
Списки
Нравится
Нравится
1:07:17
In this first episode of Neural Information Retrieval Talks, Andrew Yates and Sergi Castellla discuss the paper "Shallow Pooling for Sparse Labels" by Negar Arabzadeh, Alexandra Vtyurina, Xinyi Yan and Charles L. A. Clarke from the University of Waterloo, Canada. This paper puts the spotlight on the popular IR benchmark MS MARCO and investigates wh…
…
continue reading